Benefits of AI in Data Analytics
In recent years, the application of Artificial Intelligence (AI) in data analytics has revolutionized the way organizations analyze and interpret their data. AI, a branch of computer science that deals with the creation of smart machines capable of performing tasks that would typically require human intelligence, has provided businesses with powerful tools to extract valuable insights from massive amounts of data. This article aims to explore the numerous benefits of incorporating AI in data analytics, including its definition, key components, implementation steps, benefits, challenges, analytics reports, case studies, and future trends.
Definition:
AI in data analytics refers to the utilization of artificial intelligence techniques and algorithms to analyze and interpret large volumes of data. It involves advanced technologies such as machine learning, natural language processing, deep learning, and neural networks to uncover patterns, trends, and correlations within the data.
Key Components:
- Machine Learning: AI-powered algorithms enable machines to learn from data, adapt to new information, and improve their performance over time without human intervention.
- Natural Language Processing (NLP): NLP allows machines to understand and interpret human language, including text and audio data, facilitating the analysis of unstructured data sources such as social media posts and customer reviews.
- Deep Learning: Deep learning algorithms mimic the structure and function of the human brain, enabling the machine to automatically learn and understand complex patterns and relationships within the data.
- Neural Networks: Neural networks are interconnected systems of artificial neurons that help machines make complex decisions and predictions based on the patterns and relationships identified in the data.
Implementation Steps:
1. Data Collection: Gather relevant data from various sources, including structured and unstructured data.
2. Data Preprocessing: Clean, transform, and normalize the data to ensure consistency and eliminate any anomalies.
3. Model Development: Train AI models using machine learning algorithms on historical data to identify patterns and relationships.
4. Model Evaluation: Evaluate the performance of the AI model using appropriate metrics and fine-tune it if necessary.
5. Deployment and Integration: Implement the AI model into the data analytics pipeline and integrate it with existing systems.
6. Monitoring and Maintenance: Continuously monitor the performance of the AI model, retrain it periodically, and make necessary updates to ensure its accuracy.
Benefits:
- Enhanced Decision-Making: AI-powered data analytics enables organizations to make data-driven decisions quickly and accurately by providing valuable insights and predictions.
- Improved Efficiency and Scalability: AI automates repetitive and time-consuming tasks, allowing organizations to process large volumes of data quickly and efficiently.
- Cost Reduction: AI in data analytics eliminates the need for manual data processing and analysis, reducing operational costs.
- Better Customer Experience: AI algorithms can analyze customer data to personalize marketing campaigns, recommend products, and enhance overall customer experience.
- Fraud Detection: AI models can detect anomalies and patterns to identify fraudulent activities, saving organizations from potential losses.
- Predictive Analytics: AI models enable organizations to forecast future trends, identify potential risks, and make proactive business decisions.
- Real-Time Insights: AI-powered data analytics provides real-time insights, enabling organizations to respond quickly to changing market conditions.
- Improved Data Security: AI algorithms can monitor data for potential security breaches and quickly flag any suspicious activities.
Challenges:
- Data Quality: Accurate and reliable data is essential for effective AI-based data analytics. Ensuring data quality can be a challenge, especially when dealing with large and diverse datasets.
- Privacy Concerns: The use of AI in data analytics requires access to sensitive and personal data, raising privacy concerns that need to be addressed.
- Technical Expertise: Implementing AI in data analytics requires specialized skills and expertise. Organizations need to invest in training or hire experts to implement and maintain AI models.
- Ethical Considerations: AI algorithms must comply with ethical guidelines to avoid biased or discriminatory outcomes. Ensuring fairness and transparency in AI decision-making processes is crucial.
Analytics Report:
An analytics report generated by AI in data analytics provides valuable insights and predictions based on the analysis of large volumes of data. It presents data visualizations, statistical analysis, and key metrics derived from the data, enabling organizations to understand trends, patterns, and relationships within their data. These reports assist in identifying business opportunities, optimizing operations, and making data-driven decisions for organizational growth.
Case Studies:
1. Healthcare Industry: AI in data analytics has been used successfully in the healthcare industry to predict disease outbreaks, optimize treatment plans, and identify potential health risks in patients.
2. E-commerce Industry: AI-powered recommendations based on data analytics have significantly improved customer experience and increased sales in the e-commerce industry.
3. Financial Industry: AI algorithms have proven effective in detecting fraudulent activities, minimizing risks, and improving credit risk assessment in the financial sector.
Future Trends:
- Increased Automation: AI in data analytics will continue to automate time-consuming tasks and enable organizations to focus on more strategic and high-value activities.
- Advancements in Machine Learning: Machine learning algorithms will become even more sophisticated, enabling AI models to learn from a variety of data sources and make more accurate predictions.
- Integration with Internet of Things (IoT): The integration of AI and IoT will create opportunities for analyzing real-time data from connected devices, leading to more informed decision-making.
- Explainable AI: There will be a growing demand for AI algorithms that provide explanations for their decisions, ensuring transparency and trust in AI-powered analytics.
In conclusion, the incorporation of AI in data analytics offers numerous benefits to organizations across industries. From enhanced decision-making to improved efficiency and scalability, AI-powered data analytics has the potential to transform businesses. However, challenges such as data quality, privacy concerns, and ethical considerations must be addressed. As technology continues to advance, the future of AI in data analytics holds even greater promise, paving the way for more advanced and intelligent data-driven insights.
For more information and resources on data analytics and AI, visit the SkillsDataAnalytics website. This platform provides comprehensive training and knowledge on various data analytics techniques, including AI-powered data analytics, enabling professionals to enhance their skills and stay updated with the latest trends in the industry.
FAQs:
1. How is AI used in data analytics?
AI is used in data analytics to analyze and interpret large volumes of data, uncover patterns and trends, make predictions, and automate repetitive tasks.
2. What are the benefits of AI in data analytics?
The benefits of AI in data analytics include enhanced decision-making, improved efficiency and scalability, cost reduction, better customer experience, fraud detection, predictive analytics, real-time insights, and improved data security.
3. What are the challenges of implementing AI in data analytics?
Challenges include ensuring data quality, addressing privacy concerns, acquiring technical expertise, and dealing with ethical considerations.
4. What is an analytics report in AI-powered data analytics?
An analytics report presents insights, predictions, data visualizations, statistical analysis, and key metrics derived from the analysis of large volumes of data, assisting organizations in making data-driven decisions.
5. What are the future trends of AI in data analytics?
The future trends include increased automation, advancements in machine learning, integration with IoT, and the development of explainable AI algorithms for transparency and trust.
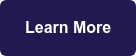